Applied Research at Moz
By pushing the frontiers of self-directed intelligence, we engineer AI agents with unprecedented levels of autonomy and adaptability.
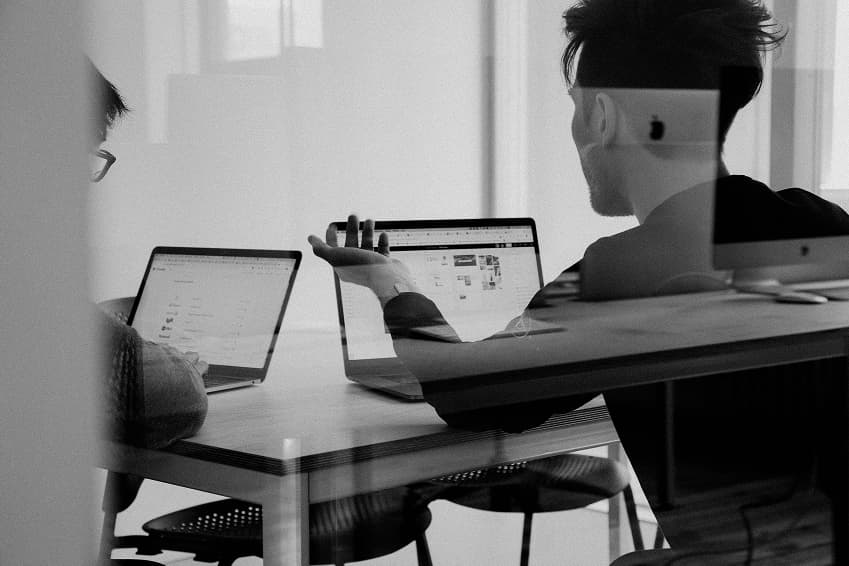
Autonomous Intelligence
Our research enhances agentic AI by employing techniques such as reinforcement learning with human feedback, self-supervised fine-tuning, and advanced prompt engineering. We refine models like decision transformers, hierarchical reinforcement learning algorithms, and action-conditioned language models to advance autonomous reasoning and adaptability. By iterating on goal-directed behavior and multi-agent cooperation, we develop AI systems that perform tasks and dynamically optimize their strategies.
Scaling Adaptive Agents
Scaling agentic AI extends beyond simply increasing model size. It involves enabling sophisticated decision-making, improved memory retention, and sustained goal pursuit through innovations in temporal reasoning, contextual awareness, and real-time interaction. We explore efficient inference strategies such as hierarchical memory management, event-driven architectures, and modular agent design. When necessary, we develop custom execution frameworks, distributed agent coordination, and optimized inference engines tailored for high-autonomy systems.
Our work in agentic AI is more than technical optimization. It is dedicated to advancing society by building systems that think, adapt, and act with purpose in dynamic environments.
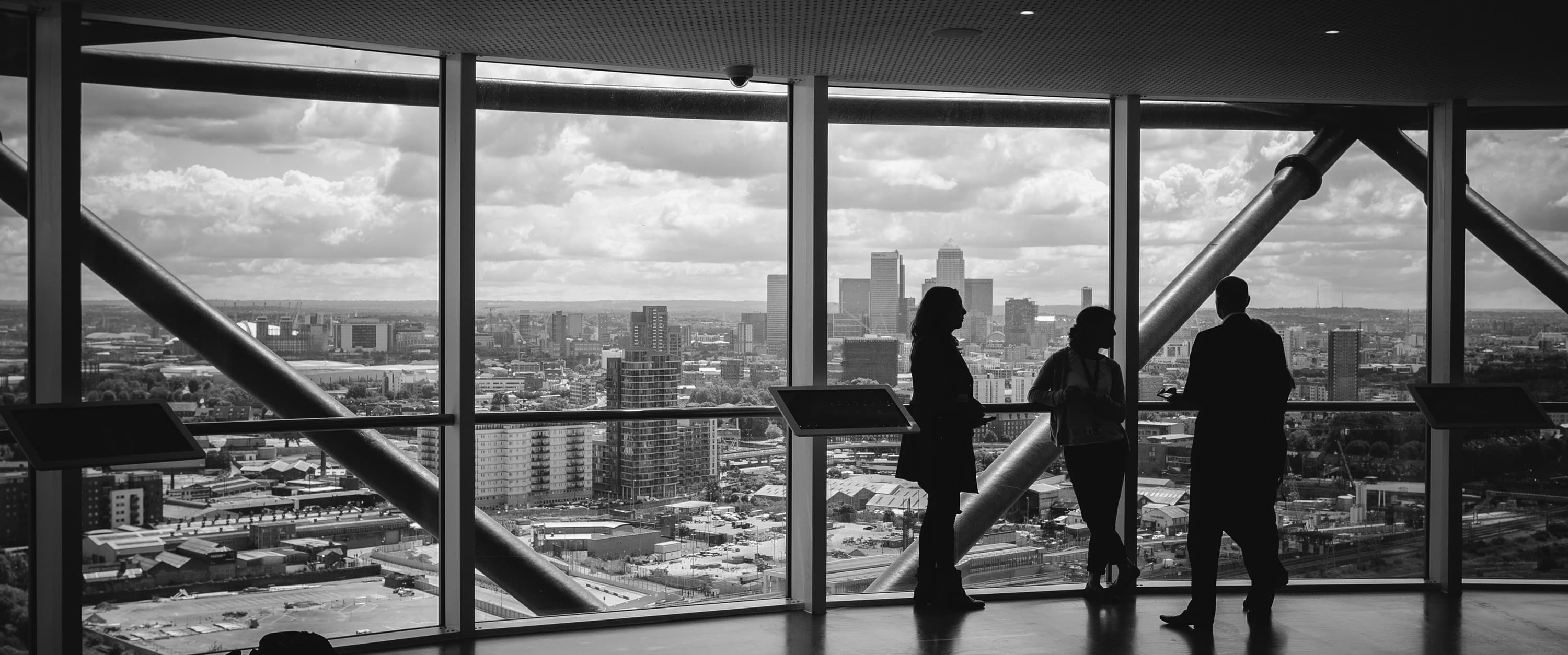